Wayne Lewis, UCLA
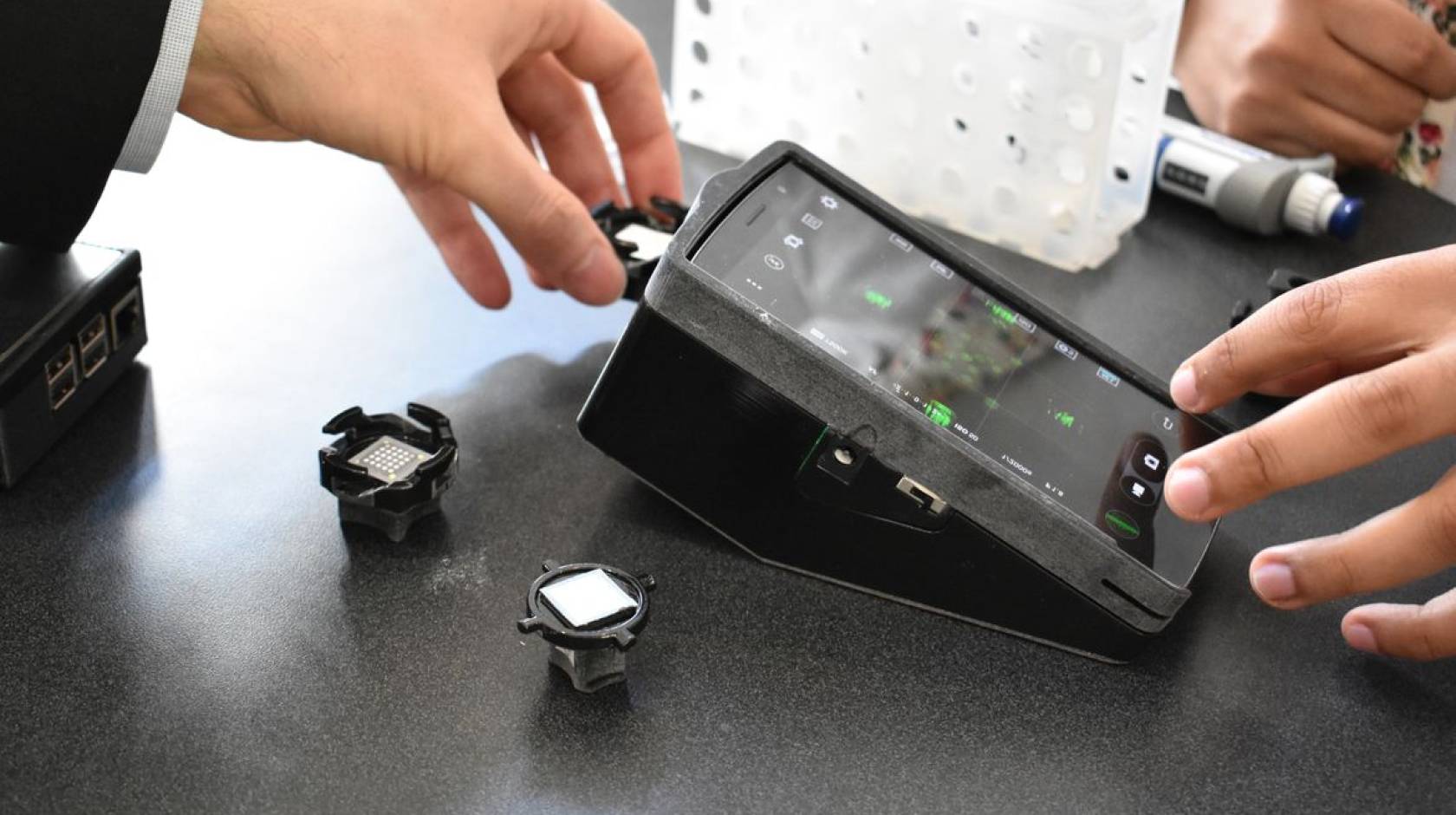
For some unlucky people, time in the great outdoors leads to Lyme disease, an illness causing head, joint and muscle pain, flu-like symptoms, fatigue, and sometimes a rash. Left untreated, those effects can turn debilitating and expand to include paralysis, inflammation of the brain and heart, and problems with memory, hearing and vision that can last for years.
Lyme disease, expected to affect more than 600,000 people in the U.S. this year, is caused by a spiral bacterium that spreads to humans through bites from infected deer ticks. The microbe provokes a complicated immune reaction that can resemble the response to other dangerous tick-borne bacteria, often making it harder for doctors to initiate the correct antibiotic treatment.
To detect Lyme disease, the Centers for Disease Control and Prevention currently recommend a two-tiered testing regimen performed at centralized labs; results take one to two weeks. Treatment with antibiotics doesn’t cure every case but can help stave off long-term disease in 80 percent to 90 percent of early-stage patients. However, according to the Bay Area Lyme Foundation, today’s testing misses seven out of 10 early-stage cases.
Members of the California NanoSystems Institute at UCLA have developed testing technology not too different from at-home kits for detecting COVID-19 infection. Results are interpreted within 20 minutes by a portable reader using artificial intelligence. In a new study published in Nature Communications, the investigators and their colleagues used patient samples to show the single AI-enhanced test is as reliable as the traditional Lyme disease testing regimen, which requires two tests.
The speed, portability and relatively low cost of the method suggests potential for same-day detection of Lyme disease — in the clinic where patients receive care.
“A lot of folks find out they have Lyme disease well after the point at which they could have been treated very easily,” said co-corresponding author Dino Di Carlo, the Armond and Elena Hairapetian Professor of Engineering and Medicine in the UCLA Samueli School of Engineering. “If we can measure rapidly, in a way that’s cost-effective and not a burden to the health system and the patient, then testing can be done more routinely. If you were out in the woods and have signs of a tick bite or other symptoms, it might be prudent to quickly test either at home or the local clinic, which could enable potential treatment earlier.”
COVID-19 home tests, in which a sample is applied to a cartridge containing special paper, offer a helpful point of comparison. The sample flows along the paper past antibodies that latch onto one disease-specific protein, which are visualized by a second set of antibodies attached to tiny gold particles measured in billionths of a meter. After a brief wait, results can be read with the human eye.
The Lyme disease detection technology goes in a different direction: A blood serum sample is dropped into a cartridge, followed by a buffer fluid, and flows vertically from top to bottom through multiple layers of sponge-like paper. One layer of paper is loaded with a set of lab-made peptides — building blocks for proteins from the Lyme disease-causing bacteria — that each detect a unique set of antibodies formed in response to that microbe. The pattern formed on the paper, which contains information about the presence and quantity of each antibody, is captured with a digital reader and analyzed by an AI algorithm that provides results. In the study, each test paper cost $3, and the reader was adapted from a $200 off-the-shelf smartphone.
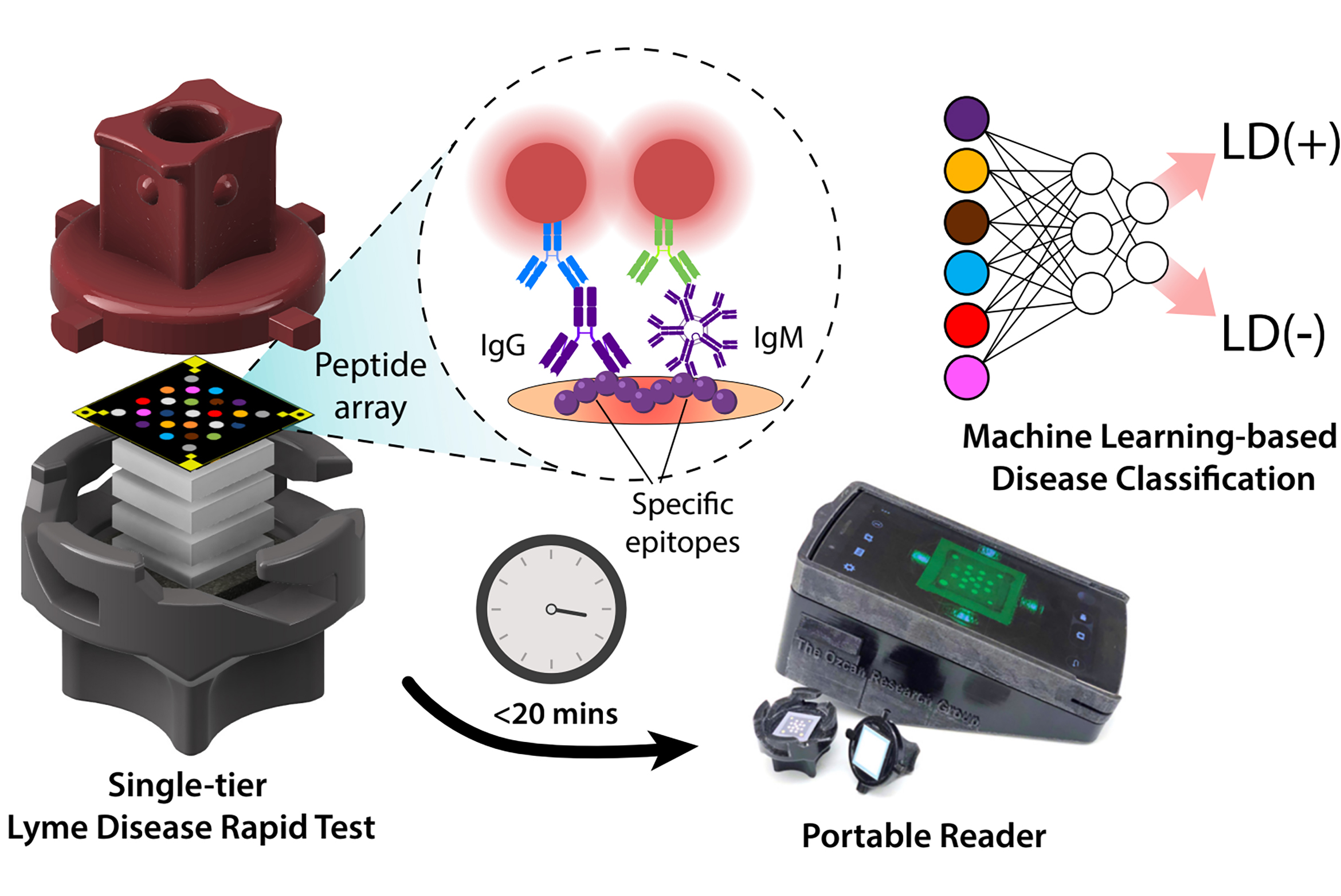
The new Lyme disease test is one of the first examples of rapid diagnostic technologies that comprehensively profile the human immune response to a disease.
“If you can quantify a panel of indicators from a single sample, you can learn a lot of interesting things regarding the patient’s condition,” said co-corresponding author Aydogan Ozcan, the Volgenau Professor of Engineering Innovation in UCLA Samueli and the CNSI’s associate director of entrepreneurship, industry and academic exchange. “In Lyme disease, we are examining a panel of immune factors that can look very different in different patients, depending on their background, where they’re from, etc. We needed AI to make sense of such a complex signal.”
The researchers trained their algorithm using several samples — including those from early-stage disease — provided by the Bay Area Lyme Foundation’s Lyme Disease Biobank and blindly analyzed their technology’s performance using new samples from the biobank. They achieved 95.5 percent sensitivity detecting Lyme disease and 100 percent specificity ruling out samples without the disease. With additional samples from the CDC, they showed their point-of-care test matched the lab-based test results very well, correctly detecting Lyme disease and differentiating it from look-alike diseases.
“AI is only as good as the data,” Ozcan said. “That’s why we worked with the Lyme Disease Biobank and the CDC to obtain well-characterized samples. Their involvement was very important for our AI to learn the patterns of immune response against the bacterium that causes Lyme disease.”
In addition to AI, synthetic peptides from Connecticut-based Biopeptides, Corp., were a key element of the new testing technology. Compared to the whole proteins used in some lab tests for Lyme disease, the peptides can be bound to more specifically, are easier to produce, and are more stable.
“The peptides are really critical,” Di Carlo said. “We want to focus on responses that are very specific to Lyme disease and not other related conditions or look-alike diseases. And at the same time, the tests can be cheaper, last longer and return fewer diagnostic errors.”
With further success, the test may take a few years to reach the clinic. The researchers are currently seeking partners to scale up their technology in order to accelerate that process. They are also working on adapting the test to use whole blood samples rather than serum, and have plans to further simplify the test format and develop a dedicated AI sample reader independent from a given smartphone.
The study’s co-first authors are UCLA’s Rajesh Ghosh, a graduate student from Di Carlo’s lab, and Hyou-Arm Joung, a postdoctoral researcher from Ozcan’s lab. Other authors are Artem Goncharov, Barath Palanisamy, Kevin Ngo, Katarina Pejcinovic, Nicole Krockenberger and Omai Garner, all of UCLA; Elizabeth Horn of the Lyme Disease Biobank; Ezdehar Ghazal and Andrew O’Kula of New York Medical College; and Paul Arnaboldi and Raymond Dattwyler of New York Medical College and Biopeptides, Corp.
The study received support from the National Institutes of Health and the National Science Foundation.