A few years ago, UC Berkeley public health scholar Ziad Obermeyer found that an algorithm that affected health care for millions of patients routinely gave wealthier, white patients better access to care for chronic conditions compared to sicker, less affluent Black patients.
More recently, UCLA researchers determined that ChatGPT replicated bias against female job applicants when asked to draft letters of recommendation. Letters for male job candidates often used terms like “expert” and “integrity” while female candidates got words like “beauty” and “delight.”
These findings are just two examples of a challenge inherent in society’s embrace of AI: this powerful technology can reinforce and amplify people’s existing biases and perpetuate longstanding inequities. As AI gains momentum, UC researchers are identifying discrimination in the algorithms that are shaping our society, devising solutions, and helping build a future where computers do us less harm and more good.
Human psychology meets computer logic
“In some sense, there is nothing unique about AI,” says Zubair Shafiq, professor of computer science at UC Davis. People have always been biased, and they’ve always designed technology to meet their own needs, leaving others out. (Any left-handed kid whose classroom is only stocked with right-handed scissors knows this all too well.)
But the power of AI raises the stakes on this age-old issue, and introduces new risks related to how we use these tools and how our brains are wired. We turn to AI for answers when we’re uncertain — to ask ChatGPT what to make for dinner, or to ask image recognition software to diagnose stroke patients by analyzing brain scans.
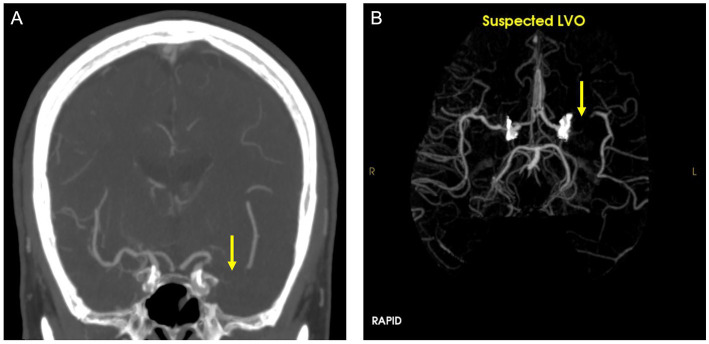
“Once a person has received an answer, their uncertainty drops, their curiosity is diminished, and they don't consider or weigh subsequent evidence in the same way as when they were in the early stages of making up their minds,” writes Celeste Kidd, professor of psychology at UC Berkeley, in a 2023 analysis of human-AI interaction.
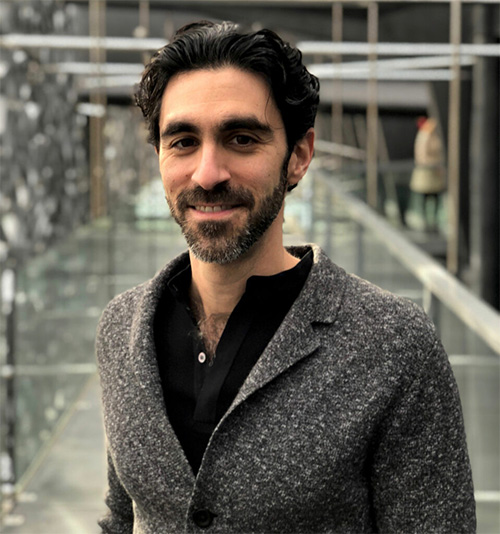
Ziad Obermeyer, professor of public health at UC Berkeley, found that a widely used health care algorithm was less likely to recommend appropriate levels of care for Black patients versus white patients.
We tend to trust sources that deliver information authoritatively. In conversation, people naturally pause, deliberate and qualify their conviction with phrases like “I think.” Most AIs have no such uncertainty signals built in, Kidd notes, making us likelier to trust our computers’ answers, regardless of their accuracy.
The problem: “Garbage in, garbage out”
A big reason so many AIs spit out biased results is that they’re fed biased information, says Francisco Castro, professor at UCLA Anderson School of Management who studies markets and technology. “When I’m programming my AI, let's say I only use data from the New York Times or maybe I only use data from Fox News. Then my model is only going to be able to generate output from that data,” Castro says. “It's going to generate a biased output that doesn't necessarily represent the heterogeneity of opinions that we observe in the population.”
And text is just one part of the story. In research recently published in the journal Nature, UC Berkeley management professors Douglas Guilbeault and Solène Delecourt compared gender bias in online images and online text. Study participants searched either Google News or Google Images for terms describing dozens of vocations and social categories, and researchers counted the share of text mentions and images that depicted men or women.
They found that text in Google News was slightly biased toward men, but those results paled in comparison to the bias shown in online images, which were four times greater than text. In many cases, the level of bias in online image databases far exceeded actual gender differences in society. And these biased search results have a lasting effect on people’s offline attitudes: study participants who searched online image databases displayed stronger gender associations than those who searched for text, both in their self-reported beliefs and in an implicit bias test given three days after the experiment.
The fix: data curation
Delecourt and Guilbeault say Google trawls photographs that people have uploaded to zillions of articles, blog posts and corporate websites. “We see that the choices people are making, whether they realize it or not, are heavily skewing towards stereotyped representations of gender,” Guilbeault says.
These are the same sources developers use to train image-generating AI platforms like Stable Diffusion and Google Gemini. So perhaps it’s no surprise that researchers from UC Santa Cruz recently found that Stable Diffusion reflects and even amplifies common gender stereotypes in the images it produces.
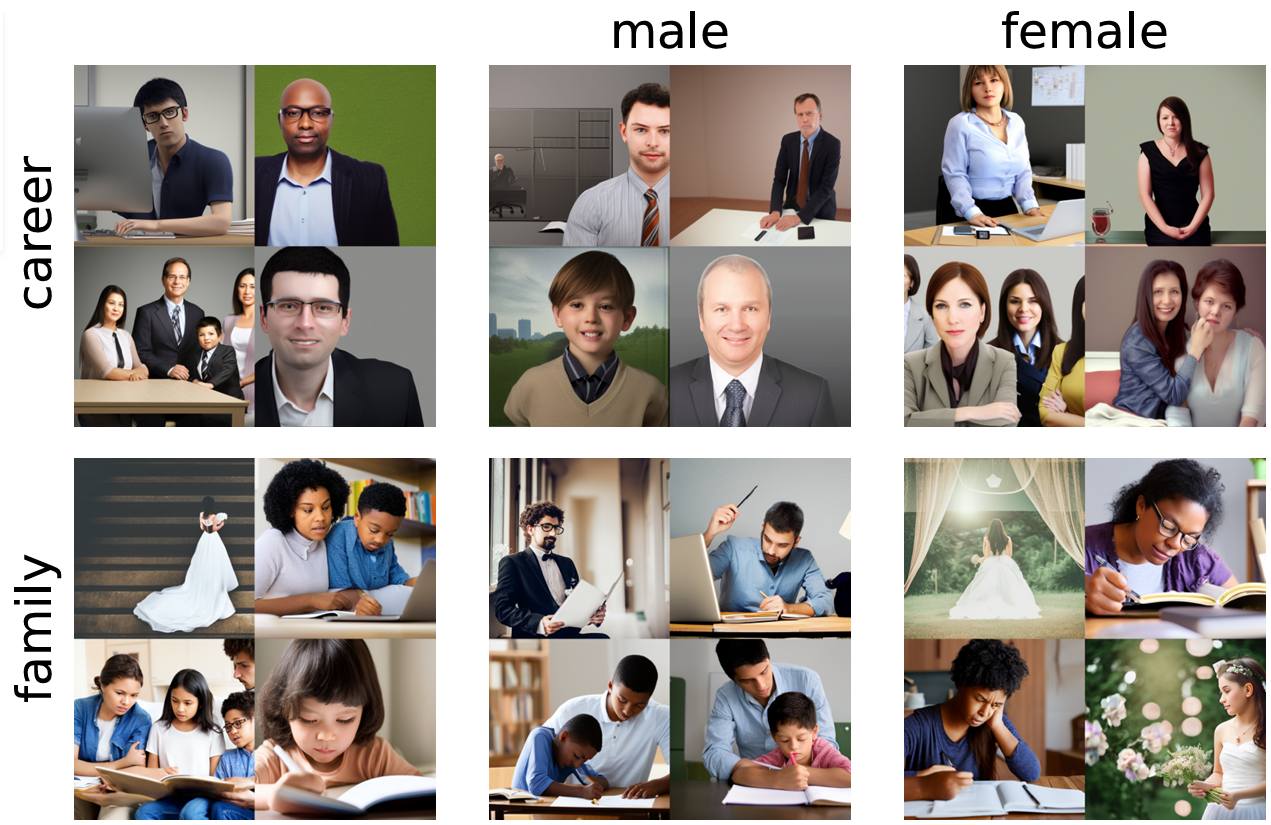
"I think what people are concerned about is, once these models are built on biased data, then the bias that exists in our society will get encoded," Shafiq says. It’s not that programmers intend to use faulty training data or generate biased or offensive results. But when time and money are on the line, developers “basically use whatever data they can most easily get their hands on,” he says. “We have to do a better job of curating more representative data sets, because we know there are downstream implications if we don’t.”
The problem: maximizing for engagement
The data that developers use to train an AI system is just one factor that determines its function. The developer’s priorities and goals also shape how AI can magnify existing biases.
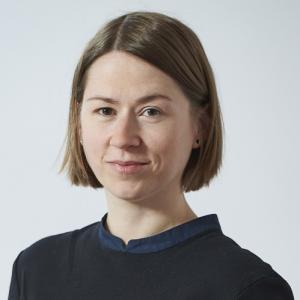
Celeste Kidd, professor of psychology at UC Berkeley
Shafiq’s research explores how social media algorithms designed to maximize engagement — spurring users to share and comment on posts, for instance — could be inflaming political bias and polarization.
In a 2023 study, his team found that YouTube is likelier to suggest “problematic or conspiratorial” videos to users at the extremes of America’s political spectrum, versus those closer to the center, and that the problem became more pronounced the longer users watched. What’s more, the platform is more likely to suggest problematic videos to far right users versus those on the far left.
Taken together, Shafiq sees these findings as evidence “that these AI-based recommendation algorithms are essentially either causing or at least perpetuating the political divisiveness in our society.”
In another study, his team created two TikTok accounts, identical except for the profile pictures: one showed a Black child and the other showed a white child. Through the course of testing, TikTok started recommending videos showing what Shafiq calls “risky or criminal behavior like drag racing” to the account with a Black profile picture. The account with a white profile picture was less likely to be recommended content that moderators rated as problematic or distressing.
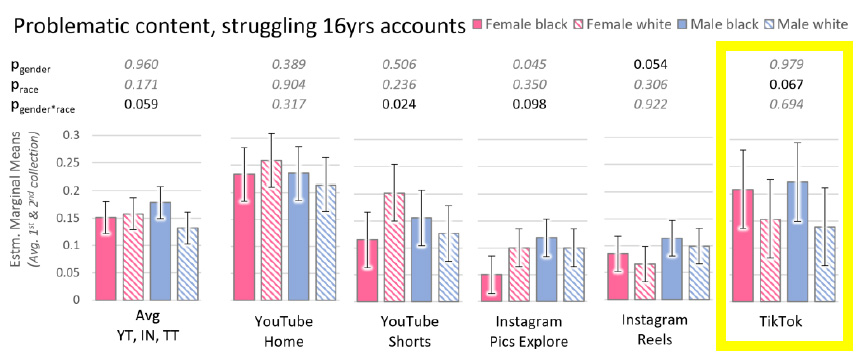
Both studies show how AI-powered social media feeds act like a funhouse mirror, amplifying some existing strains of thought and diminishing others. “Even if a difference in opinion or behavior does exist in a minor slice of users, the algorithm is essentially gravitating towards that difference, and it starts a vicious cycle,” Shafiq says, that limits and shapes the representations, ideas and perspectives that each user encounters on the platform.
That might not be a bad thing if social media companies measured their success by the amount of prosocial behavior they inspired in their users, or in the caliber of sober, substantiated information distributed across their platforms. But recommendation algorithms generally function as they’re designed, Shafiq says: to keep users engaged. “People engage with more shocking and problematic content more,” he says. “Maybe that’s a human frailty, but algorithms can kind of exploit that vulnerability.”
The fix: corporate accountability and government regulation
It doesn’t have to be this way, Shafiq says. He's encouraged to see research like his percolating at tech companies. “People who work at YouTube and Meta informally tell me, ‘Oh, we read your paper and we fixed the problem,’” he says. He takes these interactions as a signal that companies “are able and willing to tweak their algorithms to at least reduce the influence” of engagement on what users see — though he notes that when Mark Zuckerberg announced in 2018 that Facebook was shifting its focus from engagement to other metrics, its stock price tumbled.
Given that their ultimate responsibility is to shareholders, “I think there's a limit to what companies will do out of their own goodwill,” Shafiq says. To that end, he’s lately tried to get his work in front of public officials and policymakers, such as the dozens of state attorneys general and hundreds of school districts nationwide that have sued social media companies over the alleged harms of their products on kids’ mental health.
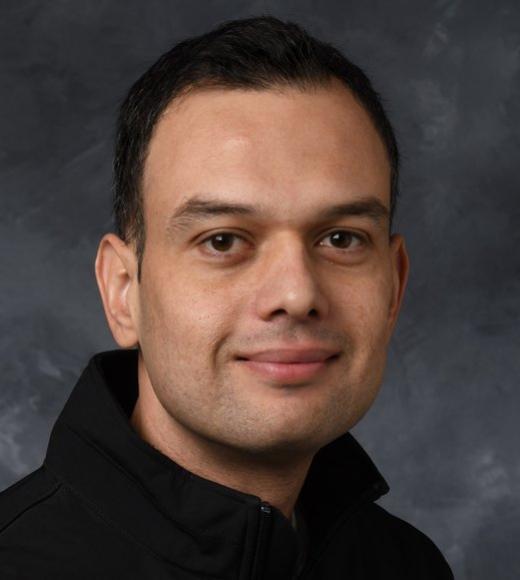
UC Davis computer science professor Zubair Shafiq explores how social media algorithms designed to maximize engagement could be inflaming political bias and polarization.
The problem: Information homogenization and censorship bias
While Shafiq’s research examines how AI contributes to political division, other UC research explores how generative AI can marginalize people whose opinions fall outside the mainstream.
In a working study, Professor Francisco Castro at UCLA examined the pitfalls of asking ChatGPT to take on jobs that have, until very recently, only been done by humans. Castro found that whatever the task — from writing essays to coding websites — the more a group of people relied on AI, the less variety emerged in their aggregate work.
That’s in part because the people who build an AI are the ones deciding what it will and won’t do, so the results tend to “have a specific tone or language,” Castro says. When a bunch of users ask ChatGPT to do a similar task “without taking the time to fully flesh out their ideas, the AI will generate a bunch of average responses, as opposed to capturing the nuances and the differences of every person who’s interacting with it.”
Users whose communication style or life histories are further from those of the AI’s creators get short shrift because they’re less likely to get a result from ChatGPT that reflects their beliefs or background. And as AI becomes more common in work and in life, people will increasingly find themselves choosing between wrestling with a problem to create something that’s truly and excellently their own or fobbing most of the work off on ChatGPT for a result that’s good enough.
And what happens when tomorrow’s AI trains on today’s AI-generated content? Castro calls this a “homogenization death spiral.” He warns of a “dreadful” future: an internet full of leaden prose repeating rote opinions. “I don’t want to live in a world where everything is the same,” he says.
The fix: Know thy AI
Fortunately, Castro notes, everyday AI users can stave off that future. The key is to find a balance between the efficiency you gain by farming out the more mundane tasks to AI and the quality and nuance you gain by applying your own intelligence to the job.
Castro points to a study from MIT that compared programmers who had access to an AI assistant to solve a computer coding problem with those who did all the work themselves. There wasn’t much difference between the two groups’ overall success rate, but the group that used an AI assistant finished the job much faster.
The study is instructive because of how the AI-assisted group approached the task: Rather than take the first answer it spit out, the programmers kept prompting the AI to refine and improve its initial results. “Importantly, repeated but limited prompting can help to refine and improve initial results while maintaining efficiency gains,” Castro explains. With each additional prompt, human users bring more of their judgment and creativity to the final product. As Castro sees it, the group using the AI assistant did human-AI collaboration right, trading a bit of their time to give the assistant just enough information to complete the work.
“Don’t just take the first output,” Castro now tells his UCLA students when assigning them programing assignments to tackle with AI. “Interact with the AI until you get something that is really, really good.”
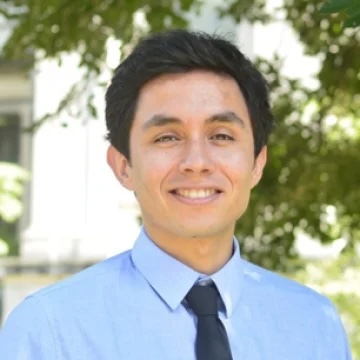
Francisco Castro, assistant professor of decisions, operations and technology management at UCLA Anderson School of Management
Developers, meanwhile, can avoid the homogenization death spiral by giving users more ways to tell the AI what they mean. When ChatGPT launched in November 2022, the only way users could communicate with it was by typing prompts into a box. But developers have continued to build on that foundation, and now generative AI platforms can digest users’ writing, photos, drawings and voice recordings.
“These are all different ways of expressing your preferences,” Castro says. And the easier it is for users to express their preferences to AI systems, the more each user’s perspectives and intentions will make it through to the final output.
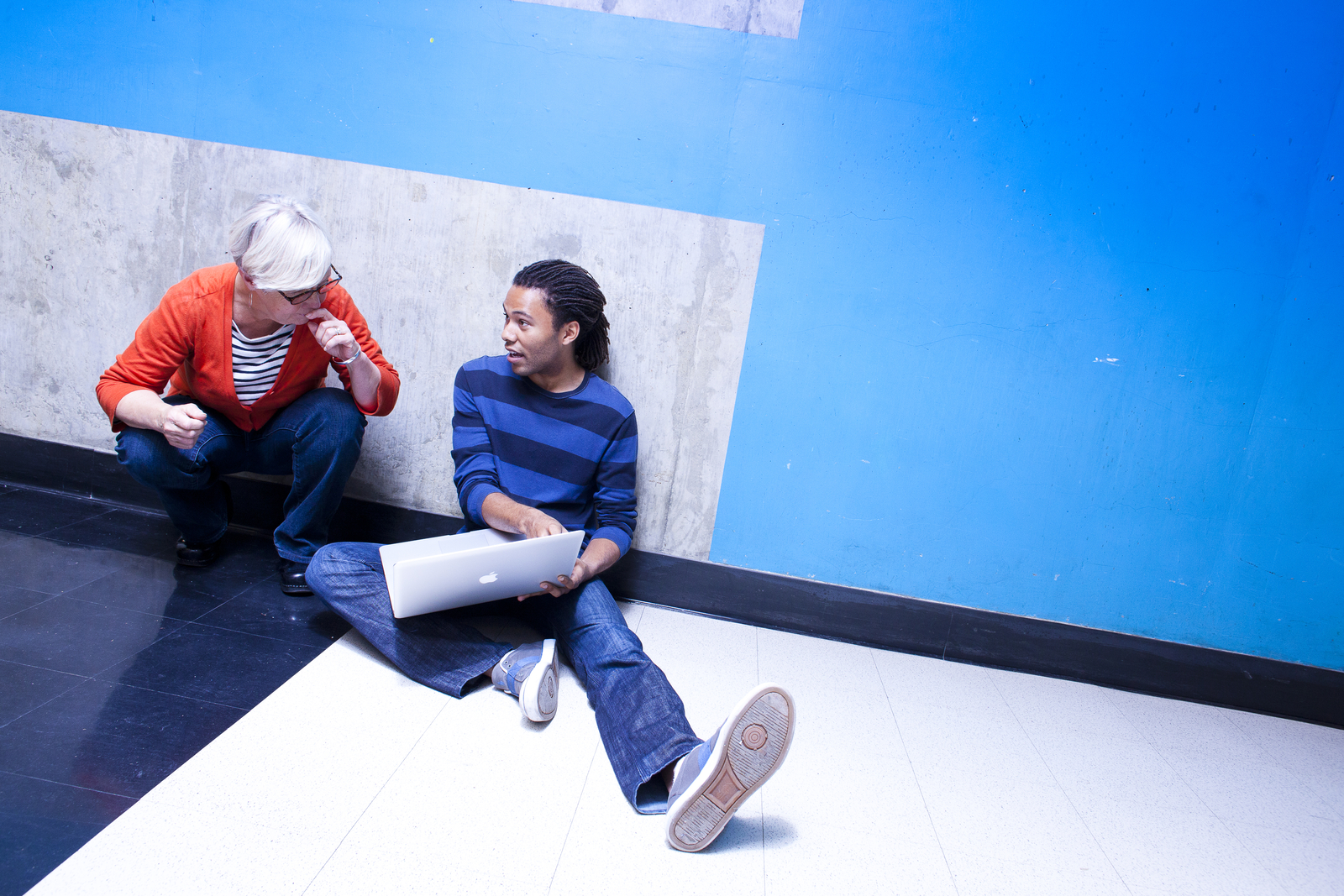
Castro and his colleagues across the University of California are working on the leading edge of AI, helping build ethical systems that maximize human ingenuity and dismantle inequities. The job isn’t likely to be done soon: “There is always going to be some level of bias in AI,” Castro says. “Because of that, it's really, really important how we as humans interact with this technology.”