Sean Nealon, UC Riverside
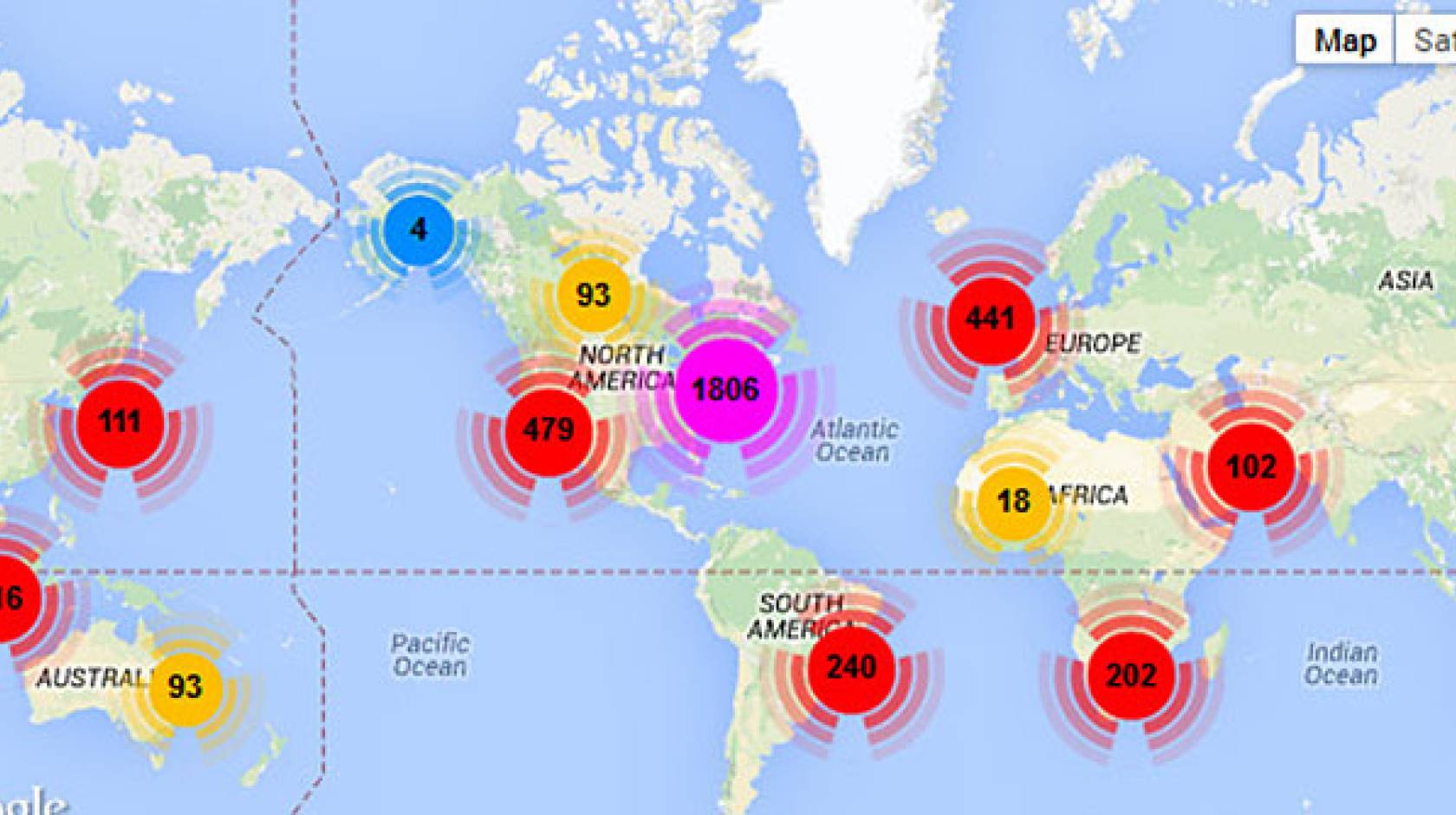
Interested in the number of tweets about the flu in recent days, weeks or months? Whether the tweets are positive or negative? How they are dispersed geographically down to the street level? Words commonly used with flu? Or even predicting of the number of tweets about the flu in the coming days?
Health Social Analytics, a website that visualizes data about health-related disorders, drugs and organizations from Twitter, news stories and online health forums, such as WebMD, does all of that.
The site is the work of Vagelis Hristidis, an associate professor of computer science at the Bourns College of Engineering at the University of California, Riverside and a team of researchers.
“This is a tool that brings the power of social and news big data to your fingertips,” Hristidis said.
The tool has applications for a wide range of groups. Government agencies could use it when preparing for a public health emergency, such as the H1N1 (swine) flu scare in 2009/10. Drug companies could use it to check the sentiment and volume of online chatter related to their drugs and these of their competitors. Media outlets could use it to track trends. Health psychologists could use to learn what keywords dominate health-related forums or which disorders have the biggest online communities.
The site is a health-specific outgrowth of a similar site, Social Predictor, which Hristidis and his group also created. Social Predictor focuses only on Twitter and news, but has a wider set of topics, including celebrities, food and real estate. The key focus of Social Predictor, as its name implies, is the prediction of future trends.
For example, if you type in “Lady Gaga” you quickly get four graphs that show number of tweets, sentiment of tweets, number of news stories and sentiment of the news stories. It defaults to the past two-month period, but the dates are adjustable.
Once the graphs are displayed, you can click on each date to a see a pop-up window that lists the most popular tweets or news stories for that day. Clicking “see more” opens a new window that displays all relevant tweets on a map.
Social Predictor also offers a tool to predict the future trends of user-input time series, such as stock prices or daily sales of a product, based on the chatter related to user-input keywords, such as a stock ticker or the name of a product.
The Health Social Analytics site breaks keywords into three health categories: disorders, drugs and organizations.
Once a keyword is selected, the page refreshes and displays a line graph in the center. On the line graph, there is a pull down menu with 16 categories that can be viewed. Most categories have to do with the number and sentiment of tweets and news stories. The user can adjust the start and end date of the graph.
In addition to the line graph, there is a Google map with color coated numbers showing high concentrations of tweets about that keyword in different parts of the world. The tweets can be broken down to the street level. The start and end date of the data collection can also be adjusted.
Finally, there is an interactive graph that looks like a spider web. It shows the selected keyword in the center and other words commonly found alongside it in tweets. The more common the word the larger the green circle next to it.
The user can also click the “Health Forums” link and see similar information about health-related Web health forum.
Health Social Analytics and Social Predictor build upon previous work by Hristidis and other researchers that used data from Twitter to help predict the traded volume and value of a stock. A trading strategy based on a model created by Hristidis and others outperformed other baseline strategies by between 1.4 percent and nearly 11 percent and did better than the Dow Jones Industrial Average during a four-month simulation.
Several current or former students contributed to the development of the two sites. They are: Michael Brevard, Shiwen Cheng, Moloud Shahbazi, William Ibekwe, Abhijith Kashyap, Ryan Rivas, Eduardo Ruiz, Shouq Sadah, Jehan Sethna and Matthew Wiley.
These projects were partially supported by the National Science Foundation, through grants IIS-1216007 and IIS-1216032.