Laura Counts, UC Berkeley
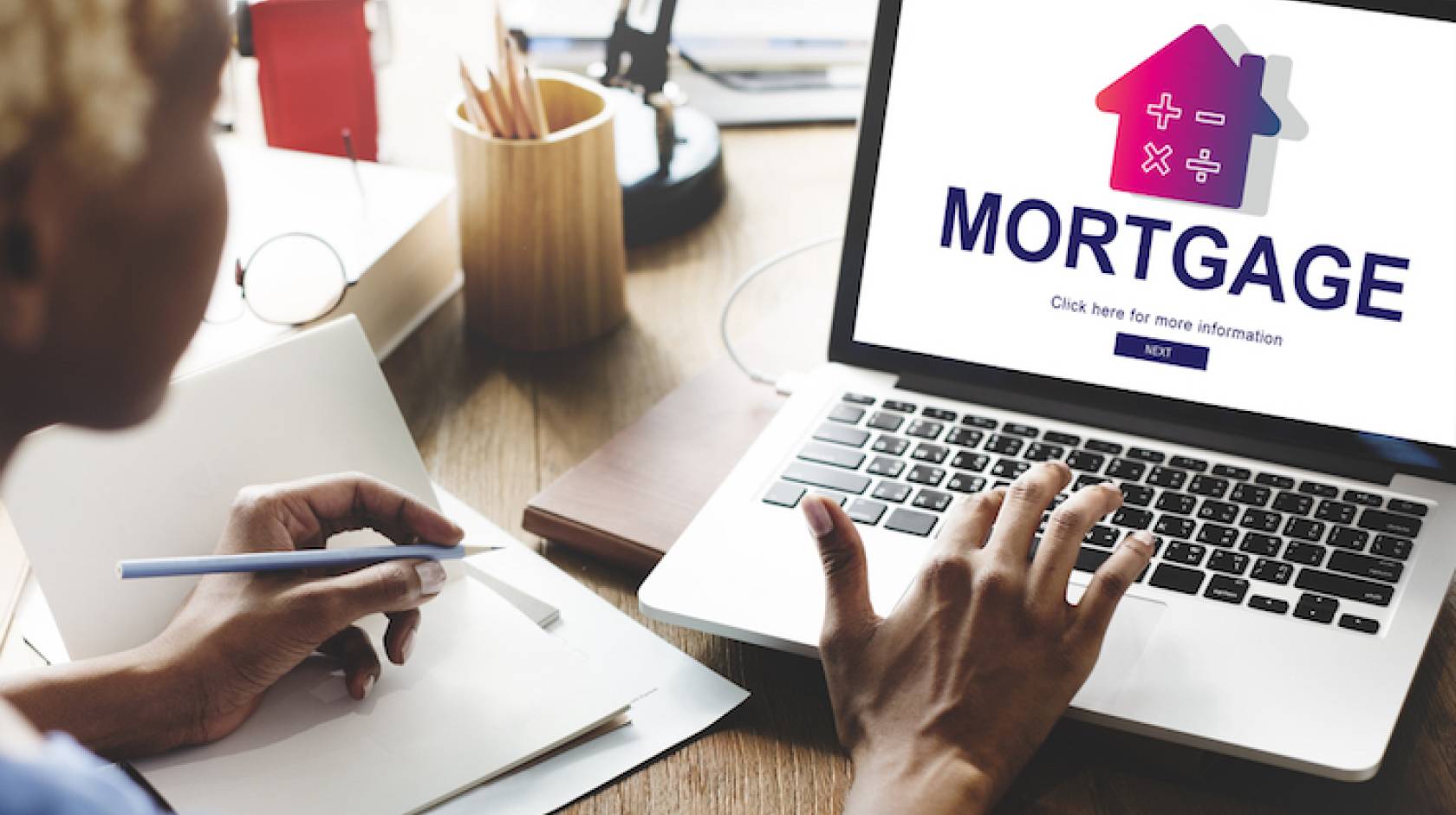
Face-to-face meetings between mortgage officers and homebuyers have been rapidly replaced by online applications and algorithms, but lending discrimination hasn’t gone away.
A new University of California, Berkeley study has found that both online and face-to-face lenders charge higher interest rates to African American and Latino borrowers, earning 11 to 17 percent higher profits on such loans. All told, those homebuyers pay up to half a billion dollars more in interest every year than white borrowers with comparable credit scores do, researchers found.
The findings raise legal questions about the rise of statistical discrimination in the fintech era, and point to potentially widespread violations of U.S. fair lending laws, the researchers say. While lending discrimination has historically been caused by human prejudice, pricing disparities are increasingly the result of algorithms that use machine learning to target applicants who might shop around less for higher-priced loans.
“The mode of lending discrimination has shifted from human bias to algorithmic bias,” said study co-author Adair Morse, a finance professor at UC Berkeley’s Haas School of Business. “Even if the people writing the algorithms intend to create a fair system, their programming is having a disparate impact on minority borrowers—in other words, discriminating under the law.”
First-ever dataset
A key challenge in studying lending discrimination has been that the only large data source that includes race and ethnicity is the Home Mortgage Disclosure Act (HDMA), which covers 90 percent of residential mortgages but lacks information on loan structure and property type. Using machine learning techniques, researchers merged HDMA data with three other large datasets—ATTOM, McDash, and Equifax—connecting, for the first time ever, details on interest rates, loan terms and performance, property location, and borrower’s credit with race and ethnicity.
The researchers—including professors Nancy Wallace and Richard Stanton of the Haas School of Business and Prof. Robert Bartlett of Berkeley Law—focused on 30-year, fixed-rate, single-family residential loans issued from 2008 to 2015 and guaranteed by Fannie Mae and Freddie Mac.
This ensured that all the loans in the pool were backed by the U.S. government and followed the same rigorous pricing process—based only on a grid of loan-to-value and credit scores—put in place after the financial crisis. Because the private lenders are protected from default by the government guarantee, any additional variations in loan pricing would be due to the lenders’ competitive decisions. The researchers could thus isolate pricing differences that correlate with race and ethnicity apart from credit risk.
The analysis found significant discrimination by both face-to-face and algorithmic lenders:
- Black and Latino borrowers pay 5.6 to 8.6 basis points higher interest on purchase loans than White and Asian ethnicity borrowers do, and 3 basis points more on refinance loans.
- For borrowers, these disparities cost them $250M to $500M annually.
- For lenders, this amounts to 11 percent to 17 percent higher profits on purchase loans to minorities, based on the industry average 50-basis-point profit on loan issuance.
“Algorithmic strategic pricing”
Morse said the results are consistent with lenders using big data variables and machine learning to infer the extent of competition for customers and price loans accordingly. This pricing might be based on geography—such as targeting areas with fewer financial services—or on characteristics of applicants. If an AI can figure out which applicants might do less comparison shopping and accept higher-priced offerings, the lender has created what Morse calls “algorithmic strategic pricing.”
“There are a number of reasons that ethnic minority groups may shop around less—it could be because they live in financial deserts with less access to a range of products and more monopoly pricing, or it could be that the financial system creates an unfriendly atmosphere for some borrowers,” Morse said. “The lenders may not be specifically targeting minorities in their pricing schemes, but by profiling non-shopping applicants they end up targeting them.”
This is the type of price discrimination that U.S. fair lending laws are designed to prohibit, Bartlett notes. Several U.S. courts have held that loan pricing differences that vary by race or ethnicity can only be legally justified if they are based on borrowers’ creditworthiness. “The novelty of our empirical design is that we can rule out the possibility that these pricing differences are due to differences in credit risk among borrowers,” he said.
Overall decline in lending discrimination
The data did reveal some good news: Lending discrimination overall has been on a steady decline, suggesting that the rise of new fintech platforms and simpler online application processes for traditional lenders has boosted competition and made it easier for people to comparison shop—which bodes well for underserved homebuyers.
The researchers also found that fintech lenders did not discriminate on accepting minority applicants. Traditional face-to-face lenders, however, were still 5 percent more likely to reject them.
Read the full paper: Consumer-lending discrimination in the era of fintech